Inteligencia artificial (IA)
AI Pulse: What's new in AI regulations?
Fall is in the air and frameworks for mitigating AI risk are dropping like leaves onto policymakers’ desks. From California’s SB 1047 bill and NIST’s model-testing deal with OpenAI and Anthropic to REAIM’s blueprint for military AI governance, AI regulation is proving to be a hot and complicated topic.
California’s proposed SB 1047 bill to mitigate AI risks sparked a storm of controversy over the summer and raised questions that still need clear answers—from how to determine AI risk to the potential impacts on AI innovation. AI model development is far from the only domain where frameworks are needed to prevent unwanted harms. This edition of AI Pulse takes a look at the topic of AI regulation and touches as well on some related issues, including how AI should be used in war and what’s going to happen to AI development when models run out of data.
Love it or Hate it, AI Regulation is Here to Stay
Even as the EU, UK, and United States were signing the first-ever legally binding treaty on AI in September 2024, experts at the European Center for Not-for-Profit Law were calling the agreement toothless—symptomatic of where we are with AI right now.
With “seize the future” champions lined up squarely against “the end is nigh” critics of AI’s breakneck evolution, legislators are in the awkward spot of having to propose regulatory guardrails with little consensus on how to evaluate risks, anticipate capabilities, or plan for the technology’s future trajectory.
This issue of AI Pulse looks at some of the challenges and thorny questions surrounding AI regulation along with the latest threat trends and the challenges ahead as AI companies run out of fresh data for training their models.
[AI News]
What’s New in AI Regulations
Out front – or out to lunch?
Hot on the heels of a star turn protecting performers from unlawful use of their digital likenesses, California lawmakers captured headlines and caught flak this summer for a more sweeping piece of proposed AI legislation, the Safe and Secure Innovation for Frontier Artificial Intelligence Models Act (SB 1047).
AI luminaries like Geoffrey Hinton and Yoshua Bengio applauded the bill, which is about to become law, while critics called the legislation off-base. Andrew Ng told Forbes that SB 1047 makes a “fundamental mistake” by regulating AI as a technology instead of focusing on specific applications. Others said it could stifle innovation.
That last worry isn’t wholly supported by real-world experience. Financial services and healthcare are famously regulated sectors and yet also leaders in AI adoption—demonstrated by recent Canadian research on AI's ability to reduce unexpected hospital deaths.
At the very least, California’s jump into the AI legislation deep end has helped highlight crucial questions other jurisdictions will need to answer if they want to follow suit—which is likely, since most Americans think AI companies should be held responsible if their technology does harm.
How many zeroes make a threat?
California decided its new law would apply to AI models that cost $100 million or more to build and are trained on at least 1026 floating-point operations (that’s one with an impressive 26 zeroes in tow). Pretty much everyone acknowledges those are imprecise measures of AI threat potential. But how should lawmakers and regulators determine AI risk? AI companies have their own frameworks, yet these also sometimes raise questions.
Case in point: OpenAI released a scorecard in September for its new o1 model. It ranked low-risk on autonomy and cybersecurity and medium-risk on persuasion and chemical, biological, radiological, and nuclear (CBRN) dangers. The company considers anything medium or lower to be deployable, though Yoshua Bengio told Newsweek that o1’s medium-risk CBRN score “...reinforces the importance and urgency to adopt legislation like SB 1047 in order to protect the public.” Persuasive behavior may be even more of a concern than dangerous information. The deceptive capabilities in o1 have also increased, raising concerns of Rogue AI.
The best bet may be for industry and government to work together on AI safety. A positive step in that direction was the late-August agreement between the National Institute of Standards and Technology (NIST), OpenAI, and Anthropic to collaborate on AI safety research, testing and evaluation.
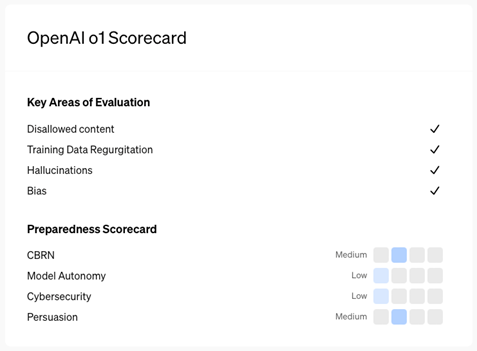
CITATION: o1 scorecard posted to the OpenAI website on September 12, 2024. Accessible at: https://openai.com/index/openai-o1-system-card/.
AI marches on to war
Most AI regulations are about preventing AI systems from doing harm. In war, the calculus is trickier: how to ensure AI-based weapons do only the right kind of harm. A recent New York Times op-ed argued the world isn’t ready for the implications of AI-powered weapons systems, describing how Ukrainian forces had to abandon tanks due to kamikaze drone strikes—a harbinger of “the end of a century of manned mechanized warfare as we know it.” (Now the Ukrainians send unmanned tanks.)
These issues were on the minds of military and diplomatic leaders who took part in the second REAIM Summit this September in South Korea. (REAIM stands for Responsible AI in the Military Domain.) The meeting yielded a Blueprint for Action outlining 20 principles for military use of AI, including that “Humans remain responsible and accountable for [AI] use and [the] effects of Al applications in the military domain, and responsibility and accountability can never be transferred to machines.”
Not all countries supported the blueprint, prompting a provocative headline in the Times of India: “China refuses to sign agreement to ban AI from controlling nuclear weapons”. The truth is more nuanced, but REAIM does underscore the vital importance of world powers agreeing on how AI weapons will be used.
CoSAI-ing up to make AI safe
The OASIS Open standards organization spun up the Coalition for Secure AI (CoSAI) this past summer as a forum for technology industry members to work together on advancing AI safety. Specific goals include ensuring trust in AI and driving responsible development by creating systems that are secure by design.
Other groups are also spotlighting best practices that industry and AI users alike can rely on for AI safety with or without legislation in place. A prime example is the Top 10 Checklist released by the Open Worldwide Application Security Project (OWASP) earlier this year, which outlines key risks associated with large language models (LLMs) and how to mitigate them.
One current top-of-mind concern for many observers is the deceptive use of AI in elections, especially with the U.S. Presidential campaigns speeding toward the finish line. Back in March, nearly two dozen companies signed an accord to combat the deceptive use of AI in 2024 elections including Adobe, Amazon, Anthropic, Arm, ElevenLabs, Google, IBM, Inflection AI, LinkedIn, McAfee, Meta, Microsoft, Nota, OpenAI, Snap, Stability AI, TikTok, Truepic, X, and Trend Micro—another example of the power of (and need for) collective action on AI safety.
[AI Threat Trends]
AI Threat Trends
DOJ nabs Russian Doppelganger domains
On September 4 the U.S. Department of Justice announced its seizure of 32 internet domains being used to “covertly spread Russian government propaganda with the aim of reducing international support for Ukraine, bolstering pro-Russian policies and interests, and influencing voters in U.S. and foreign elections....” Those activities were all part of an influence campaign dubbed ‘Doppelganger’ that broke U.S. money laundering and criminal trademark laws.
U.S. authorities remain on high alert against disinformation, manipulation, and the deceptive use of AI to skew November’s Presidential election results. According to Fox News, U.S. Attorney General Merrick Garland is also taking aim at Russia’s state-controlled Russia Today (RT) media outlet, which Meta announced it was banning from Facebook and Instagram on September 17 due to alleged foreign interference.
“Let me check my riskopedia...”
This August, MIT launched a public AI Risk Repository to map and catalogue the ever-growing AI risk landscape in an accessible and manageable way. The current version enumerates more than 700 risks based on more than 40 different frameworks and includes citations as well as a pair of risk taxonomies: one causal (indicating when, how and why risks occur) and the other based on seven primary domains including privacy and security, malicious actors, misinformation, and more.
MIT says the repository will be updated regularly to support research, curricula, audits, and policy development and give the full range of interested parties a “common frame of reference” for talking about AI-related risks.
Grok AI feeds on X user data for smart-aleck ‘anti-woke’ outputs
X’s Grok AI was developed to be an AI search assistant with fewer guardrails and less ‘woke’ sensitivity than other chatbots. While decidedly sarcastic, it has turned out to be more open-minded than some might have hoped—and controversial for a whole other reason. This summer it surfaced that X was automatically opting-in users to have their data train Grok. That raised the ire of European regulators and criticism from folks like NordVPN CTO Marijus Briedis, who told WIRED the move has “significant privacy implications,” including “[the] ability to access and analyze potentially private or sensitive information... [and the] capability to generate images and content with minimal moderation.”
[AI Predictions]
What’s Next for AI Model Building
AI is heading for a major data drought
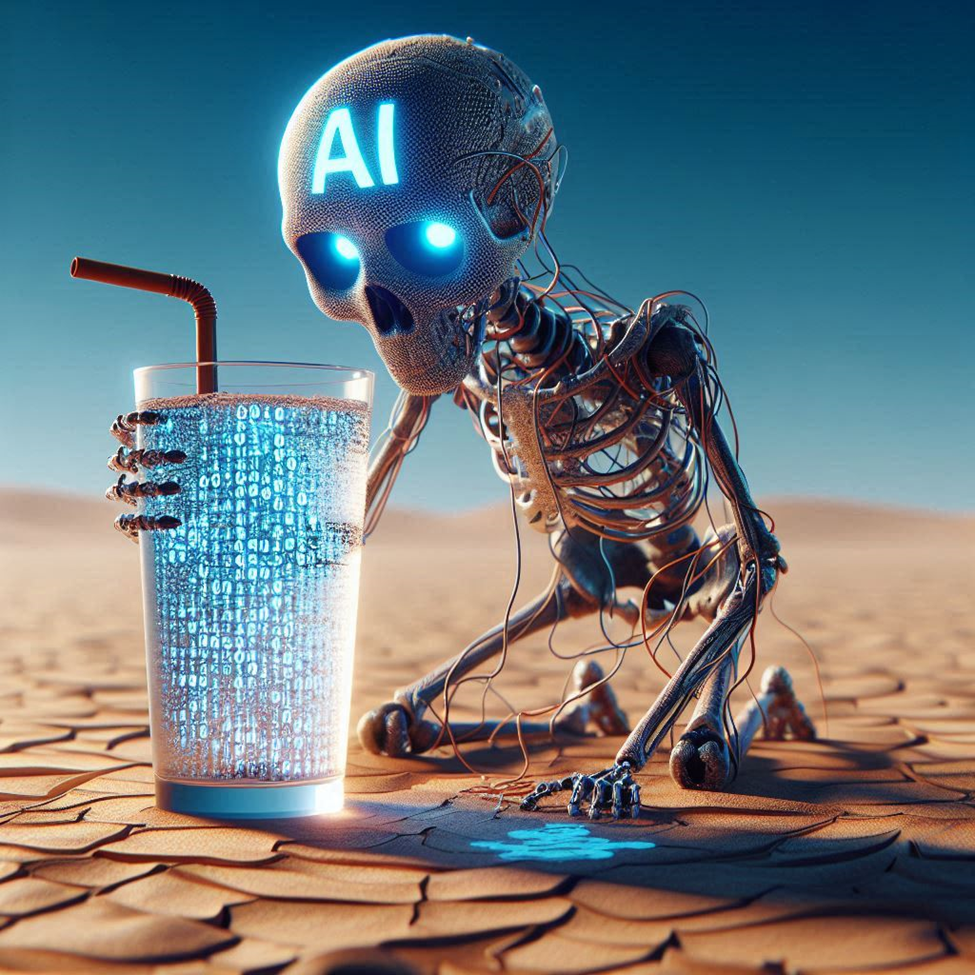
Source: Bing: An AI LLM in a desert. It is thirsty with wires showing and skeletal to represent dehydration. It is holding a glass full of data that shimmers with more noticeable ones and zeros. The head of the skeleton is labeled 'AI' and has glowing blue eyes. The ground is parched and dry.
Grok AI isn’t the only platform caught up in controversy over how it captures data. At the start of September, Clearview AI got hit with a $33 million fine for compiling an illegal database of 30 billion images to fuel facial recognition services for law enforcement.
Part of the problem is that AI companies are under pressure to find fresh sources of data for model training. The bigger the models get, the more data they need, but many websites are getting fiercer about protecting their content.
According to a paper published by dataprovenance.org, a “rapid crescendo of data restrictions from web sources” in 2023–2024 “will impact not only commercial AI, but also non-commercial AI and academic research.” As well, many generative AI models are now training on content created by earlier versions of themselves—risking what Nature has termed “model collapse”. Fresh, human data is essential to high-quality outputs.
To scale or not to scale, that is the question
Given the threat of data drought and the computational intensiveness of AI models, some companies are experimenting with smaller, lighter-weight models. NVIDIA’s Mistral-NeMo-Minitron 8B is a “width-pruned” version of the company’s NeMO 12B base model that’s “small enough to run on an NVIDIA RTX-powered workstation while still excelling across multiple benchmarks for AI-powered chatbots, virtual assistants, content generators and educational tools,” according to a recent NVIDIA blog.
Microsoft is also creating smaller models. A recent post on X suggests a local version of Copilot is now shipping with the RWKV model, which has a recurrent neural network (RNN) architecture instead of the de facto Transformer standard, RWKV has, making it faster and less power-hungry. (RWKV has also released a free, Transformer-based model sized specifically for math and coding problems.)
AI’s power problem
Beyond its appetite for data, AI is also a voracious consumer of electricity. That was front-and-center in September when the U.S. Senate Committee on Energy and Natural Resources queried the Department of Energy (DOE) on AI’s power needs. The director of DOE’s Office of Critical and Emerging Technologies said that since 2010 the number of computations for AI models has doubled every six months. The department is looking to expedite permitting processes to ensure sufficient energy resources.
Elon Musk, at least, isn’t waiting on that. He claims he’s built the world’s largest data center in Memphis, Tennessee, with 100,000 GPUs online and 100,000 more coming soon. Instead of waiting for power hookups, he installed 20 natural gas turbines with no permits.
Yet even if Musk and others push ahead with buildouts of massive infrastructure, they will still need to solve the data-for-training problem—which as we’ve seen may be easier said than done.
Postscript: Agentic AI Redux
Our August AI Pulse focused on the coming of ‘agentic AI’—platforms and solutions powered by autonomous AI agents. Salesforce jumped on that bandwagon this past month with the announcement of 'Agentforce', described by Chair and CEO Marc Benioff as “a revolutionary and trusted solution that seamlessly integrates AI across every workflow, embedding itself deeply into the heart of the customer journey.” The company’s news release declares the goal of empowering a billion agents with Agentforce by the end of 2025, stating, “This is what AI is meant to be.”
More perspectives from Trend Micro
Check out these additional resources:
- [Video] AI Regulation Challenges
- [Newsletter] Navigating the Dark Side of AI
- Identifying Rogue AI
- [Video] Xday 2024: The Unofficial AI Survival Guide