Artificial Intelligence (AI)
AI Pulse: Sticker Shock, Rise of the Agents, Rogue AI
This issue of AI Pulse is all about agentic AI: what it is, how it works, and why security needs to be baked in from the start to prevent agentic AI systems from going rogue once they’re deployed.
Cut the Chatter, Here Comes Agentic AI
Major AI players caught heat in August over big bills and weak returns on AI investments, but it would be premature to think AI has failed to deliver. The real question is what’s next, and if industry buzz and pop-sci pontification hold any clues, the answer isn’t “more chatbots”, it’s agentic AI.
Agentic AI transforms the user experience from application-oriented information synthesis to goal-oriented problem solving. It’s what people have always thought AI would do—and while it’s not here yet, its horizon is getting closer every day.
In this issue of AI Pulse, we take a deep dive into agentic AI, what’s required to make it a reality, and how to prevent ‘self-thinking’ AI agents from potentially going rogue.
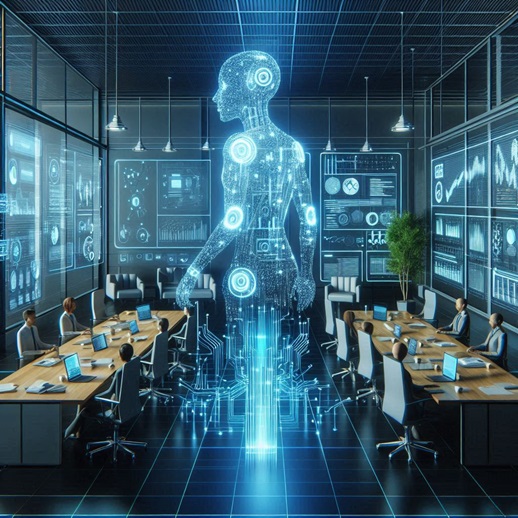
What’s new: Agentic AI
Revolution ain’t cheap
Big tech investors sprouted a fresh crop of grey hairs this summer as companies like Microsoft, Meta, and Google acknowledged how much cash they’ve been pouring into AI development and infrastructure. Reports say OpenAI alone could lose $5 billion this year keeping up its trailblazing efforts. While name-brand CEOs admit the “how much” of AI spending may be too much right now, they’re quick to insist underspending would be even riskier.
Those reassurances haven’t kept some observers from trotting out the B-word (“bubble”) with respect to generative AI. But questioning the potential of GenAI to live up to the hype is a bit like imagining Alexander Graham Bell’s contemporaries doubting his prototype telephone would ever transform planetary communication: not in that specific form, no; but as a concept, absolutely. Current large language models (LLMs) may not be able to reason—resulting in flaws like the “reversal curse” of not being able to infer relationships between facts—but such drawbacks will eventually be overcome. Solving them will usher in the era of agentic AI.
‘Upsmarting’ AI
Today’s GenAI tools are producers: they generate text, images, code, analyses. But the real power of AI will come from thinking and doing—solving problems and taking actions. McKinsey & Company posted a paper at the end of July explaining how GenAI agents will drive the push from “information to action” by adapting to dynamic situations (instead of following rules-based logic), responding to natural language prompts, and interacting with existing software platforms. Each agent will essentially be an autonomous thinking unit that, in the best-case scenarios, helps execute workflows more effectively, with better, more accurate outcomes.
Citing AWS guidance, ZDNET counts six different potential types of AI agents:
- Simple reflex agents for tasks like resetting passwords
- Model-based reflex agents for pro vs. con decision making
- Goal-/rule-based agents that compare options and select the most efficient pathways
- Utility-based agents that compare for value
- Learning agents
- Hierarchical agents that manage and assign subtasks to other agents
Agentic AI’s emphasis on automation and decision support “has the potential to augment human capabilities rather than replace them,” according to an August Forbes article—a possible silver lining given the longstanding concerns that AI will cause job loss and economic displacement.
Are we there yet?
If the agentic model shifts AI from making to doing, it will also shift humans from being tool users to ‘goal staters’—tasking AI systems with achieving desired outcomes. How close are we to seeing this happen? Back in July, Bloomberg reported on OpenAI’s five-step pathway to artificial general intelligence. Conversational chatbots sit at level 1, ‘reasoners’ at level 2 and agents at level 3. The final two stages are ‘innovators’ and AI that can do the work of an organisation. AI companies are currently just getting to level 2, but agentic AI is the next stop on the roadmap, and when we get there it’s likely to dwarf the LLM wave in scale and duration.
One route to getting there builds on the precedents of game-playing AI. When AI reasoning expert Noam Brown joined OpenAI in 2023, he posted on LinkedIn that generalising methods of AI self-play could lead to “LLMs that are 1,000x better than GPT-4.” While the ability of AI to ‘ponder’ problems and make inferences adds processing time and cost, Brown suggested the trade-offs would be worth it, especially in domains like cancer care. That said, AI companies clearly have their work cut out for them—which may be a good thing, since there are vital cybersecurity questions to answer first.
AI threat trends
“You’re gonna need a bigger boat.”
Roy Scheider’s famous line when he first sees the giant shark from Jaws echoes what many forward-looking security experts are saying about the cyber risks of agentic AI. Today by far the biggest AI-related threats are fraud, data privacy, and careless use. But once agents start making decisions and implementing actions on their own—potentially in ways that aren’t auditable or retraceable—there’s a very real risk they could go rogue, acting against the interests of their creators, users, or humanity in general.
To prevent that, AI platforms need both internal guardrails and external containment: embedded ‘do no harm’ principles combined with multilayer zero trust security and defence-in-depth approaches, as detailed in a recent Trend Micro blog series on rogue AI.
A challenge will be to confirm that AI systems are meeting safety expectations. TechCrunch reports many AI safety assessments fall short, with one study finding “current evaluations... [are] non-exhaustive, can be gamed easily and don’t necessarily give an indication of how models will behave in real-world scenarios.” Building in controls and means of verifying safety will be key to ensuring safe use of agentic AI.
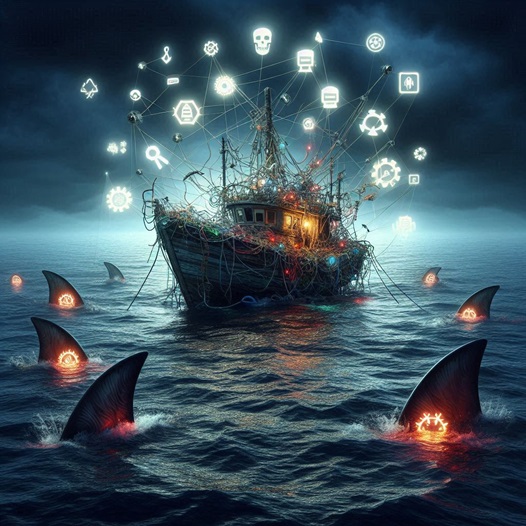
Fakeouts at the checkout
Fraud continues to be front-and-centre amongst the threat trends we’re tracking for AI Pulse. Even without technologies like agentic AI, scammers’ capabilities are advancing by leaps and bounds. While it’s hard to say where AI is being used without staring over an attacker’s shoulders, it’s clearly playing a role. The volume and sophistication of phishing and business email compromise (BEC) attacks have both increased, suggesting some kind of capacity boost. Trend Micro’s sensor protection network is picking up artefacts that appear to have been created by generative AI, and fake domains and websites also seem to be using the natural language and multimodal content creation capabilities of LLMs.
Just a couple of weeks ago, ConsumerAffairs posted an article complete with a “spot the fake Amazon page” quiz on how millions of shoppers are getting duped into phoney transactions using websites that look legitimate. The piece notes the use of dirt-cheap “phish kits” criminals can use to launch readymade scam sites with ease. It also cites a Memcyco study that found four fake Amazon sites in a single scan, adding that in 2023 Amazon spent more than $1.2 billion and had a team of 15,000 people focused on fraud prevention.
10,000+ athletes. 200+ countries. 140+ cyberattacks.
As predicted in our June AI Pulse, the summer’s Olympic Games in Paris did indeed see a wave of cyberattacks—more than 140, all told. According to Anssi, the French cybersecurity agency, the Games themselves weren’t affected. Government organisations and sporting, transportation, and telecommunications infrastructure were the main targets, with a third of attacks causing downtime and half of those attributed to denials-of-service.
The most notable incident was a ransomware attack on the Grand Palais—which did have a role in hosting the Games—and dozens of French museums, though the agency said Olympics-related information systems weren’t affected.
Swimming in a sea of AI slop and slime
We wrote a fair bit about deepfakes in our first issue of AI Pulse, but they’re not the only kind of potentially harmful AI-generated content. The Washington Post ran a mid-August story about the proliferation of images on X created with the AI image generator, Grok. While there’s nothing inherently malicious about Grok, the article raised concerns about its lack of guardrails, citing posts featuring Nazi imagery.
AI is also responsible for growing volumes of nuisance content known colloquially as ‘slop’—material that’s meant to look like it was made by people and blurs the lines between legitimate, valuable content and misleading, time-wasting junk. Worse still is the outright slime of misinformation, which a spring 2024 study found is too often and too easily regurgitated by AI chatbots. According to the NewsGuard website, 10 leading chatbots when put to the test repeated false Russian information about a third of the time, raising concerns about sources of truth for perspective-seekers in a high-stakes election year.
What’s next with agentic AI
Please, not another @*#&%*!! chatbot!
Claims that GenAI is failing to deliver on its promise are shortsighted to say the least, predicated on the confusion that LLMs are somehow the be-all and end-all of artificial intelligence. They’re not. If interest has plateaued—which the big players’ second-quarter results seem to show—all it proves is that the world doesn’t need another chatbot. The real demand is for what’s coming next: adaptive problem solving.
That capability won’t just come from building bigger LLMs. It requires a deeper solution engineering approach and the development of compound or composite systems.
Divide and conquer
As the name suggests, composite systems have multiple components that work together to perform higher-order tasks than a single statistical model can manage on its own. The hierarchical version of agentic AI captures some of this concept by engaging and coordinating multiple agents to do different things in pursuit of a common goal.
According to the Berkeley Artificial Intelligence Research (BAIR) team, composite systems are easier to improve, more dynamic than single LLMs, and have more flexibility in terms of meeting user-specific performance (and cost) goals. They are also arguably more controllable and trustable, since instead of relying on ‘one source of truth’ (a lone LLM), composite outputs can be filtered and vetted by other components.
The ultimate version of a composite system would be an AI mesh of agents that interact both inside the system itself and also ‘talk’ to other agents across organisational boundaries.
Swinging the cloud pendulum
InfoWorld points out that forms of agentic AI already exist in phone-based personal assistants to cars and household environmental controls. As organisations adopt these kinds of technologies, many are choosing to adapt their approach to infrastructure—blending on-premises, on-device, and in-cloud AI for flexibility and performance. Agents can be expected to pop up everywhere from wearable devices to laptops and data centres. Creating enclaves of safety and trust in this interconnected ecosystem requires attention as the AI mesh builds up.
Caging the bear
Moving to a composite framework in which LLMs and agents communicate and work together will lead to better AI outcomes. But as noted above, keeping this kind of AI safe and secure requires a ‘bigger boat’ or, in the words of AI guru Yoshua Bengio, a ‘better cage for the AI bear’. Basically, this comes down to a question of alignment: is the AI system fulfilling its desired objectives or pursuing undesired ones? (This is complicated by a further question: whose objectives, and which ones are most desirable?)
Today it seems there’s more push to get to the next level of AI reasoning than there is to build security into AI. That needs to change or we will end up with rogue AI models that seek to achieve goals we didn’t give them—and aren’t in our best interest.
More agentic AI perspective from Trend Micro
Check out these additional resources: